Quality control during the last 20 years - What has changed and what role does artificial intelligence play by now?
Episode 1
Author: Hanna Nennewitz

After my brief introduction, we will now get started with the actual content of this blog. We'll start by looking at how the various visual quality control solutions have changed over the last 20 years. What do these solutions look like? When did artificial intelligence become relevant? And most importantly, how does artificial intelligence differ from classical solutions?
To find out more, I talked to Dr. Wieland Brendel. He is co-founder of Maddox AI and contributes as Lead Machine Learning Advisor to the continuous product development of Maddox AI. In addition, Wieland is also involved in research. He has been conducting research on AI since 2014 and recently started leading a research group on Robust Machine Learning at the Max-Planck Institute for Intelligent Systems in Tübingen.
At the beginning of the timeline of visual quality control is, of course, as with all things, the human being. There were and still are inspectors at the assembly line who check the individual parts for defects. The first automation step in visual quality control came about 30 years ago with rule-based systems. Wieland explains it as follows: “When I talk about a rule-based system, I’m talking about a system that, at its core, is based on rules defined by people.”
Simplified, the programming process looks something like this: If, for example, you want to check the number of punched holes in a sheet of metal, the first thing a group of programmers does is to think about how exactly the system recognizes holes in a sheet of metal. These considerations lead to rules that are used to program the system. Rules can be for example like this: If you see 10×10 white pixels, then there is a hole. There should be 20 holes on each sheet. For the system to work well, the rules must be clear and always 100% valid. After programming, the system can immediately start classifying objects without seeing sample images first. The classification is based on the always valid rules previously established by the human being.
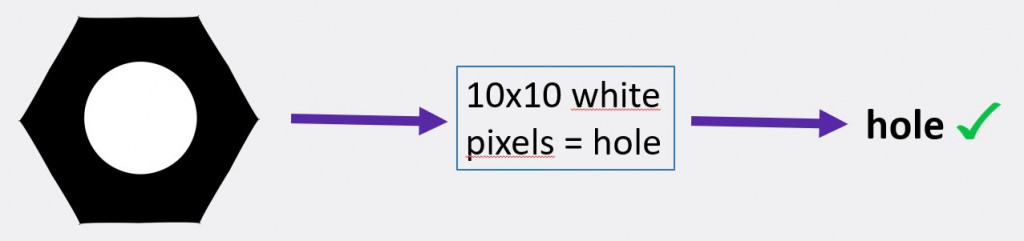
"Machine learning replaces the rules we wrote down for rule-based systems with a learning process."
However, inspecting more complex problem in visual quality control poses a problem for rule-based systems. For rule-based systems to work well, the rules must always apply one hundred percent of the time. Such rules are easy to establish for holes, but not for scratches, for example. These show a high variability due to different depth or different appearance. This makes it difficult to establish unambiguously valid rules. AI-based or more precisely ML-based systems, such as Maddox AI, can deal with these complexities and variability much better. But what is meant by AI? And what is hidden behind the abbreviation ML?
Wieland explains it best again: “AI is a broad generic term that unites many different systems. There is not really a uniform definition. Roughly speaking, artificial intelligence describes everything where machines take cognitive abilities or tasks from humans. In recent years, the term artificial intelligence has become even more narrow. It is often used synonymously with machine learning (ML), which is actually classically only a subset of artificial intelligence. But machine learning is THE field why we talk about AI at all today.”
The bottom line with machine learning is this: The machine goes through a learning process. Solving the task is no longer given to the machine by defining exact rules beforehand, but machine learning replaces this rule-making with a learning process. The machine is given examples through which it learns the rules for solving the task itself. This makes the decisive difference and advantage over rule-based systems. When humans try to set rules for complex issues, it often doesn’t work out well. They forget things or it is very difficult to put the facts into words at all, let alone to think of every important detail. Let’s take the example of scratches again: Try to think of rules by which one always recognizes a scratch. You will quickly come to the conclusion that this is almost impossible because scratches can be so different. You simply cannot write down a rule that applies one hundred percent of the time. But if the system learns for itself, as in machine learning, you can circumvent this problem. The system makes up the rules itself and the human no longer has to describe anything and, accordingly, cannot forget anything.
But what does the learning process in machine learning look like? At the beginning, there are many images. On these images it is, for example, precisely labeled: this is a scratch, this is another scratch, and this is a dent. The system is shown not just ten, not a hundred, but sometimes a thousand, ten thousand or even millions of these labeled images. During the training process, the system learns the desired function and adapts to the training data again and again. And at some point, the machine understands and recognizes on new images: That’s a scratch, that’s a crack, that’s a bump, etc.
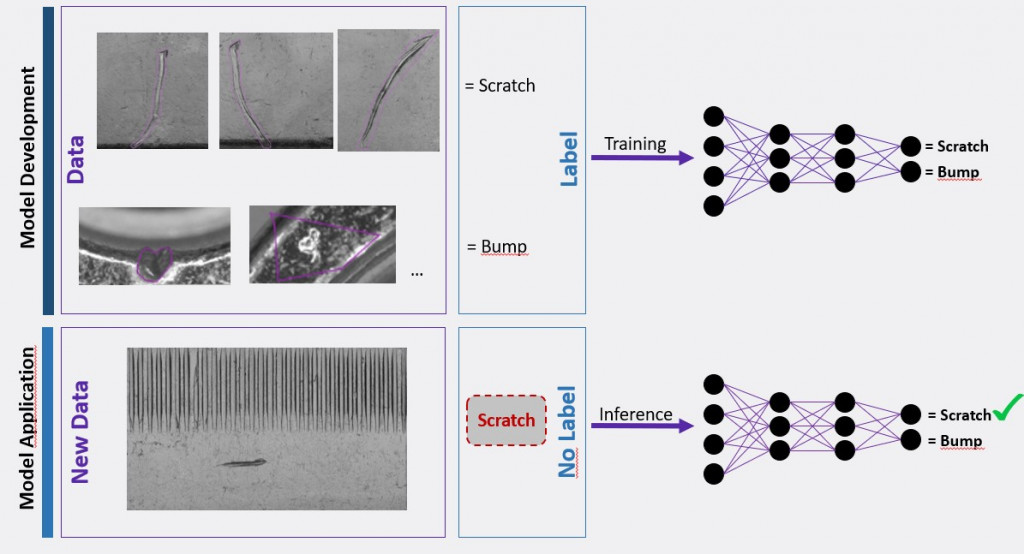
"The fact is, however, it works, and in many cases it works better than the human examiner."
According to Wieland, it is not entirely clear what exactly happens within the system: “We don’t know what the system learns exactly. We understand it better than we did a few years ago, but essentially it still represents a black box. We don’t really know how the models recognize or classify objects. We may just have to live with that, too. As a human, I don’t really understand how I recognize a scratch either. It’s unsatisfying that I don’t know that about the machine either, but the fact is, it works, and in many cases it works much better than the human inspector.”
Machine learning, then, means that the machine itself undergoes a learning process and can thus handle much more complex use cases than rule-based systems. As a result, ML-based systems like Maddox AI are opening up entirely new possibilities in quality control for manufacturing companies. I’ll turn to a more detailed description of these possibilities and what the advantages and disadvantages of rule-based systems and ML-based systems are in the next blogpost.
However, if you want to experience the new possibilities Machine Learning offers for visual quality control right away, feel free to get in touch with us. Our team will be happy to take the time to explain the application of Maddox AI to you in more detail and to check the possible installation in your company.
Visual Quality Control - Rule-Based Systems vs. ML-Based Systems
Episode 2
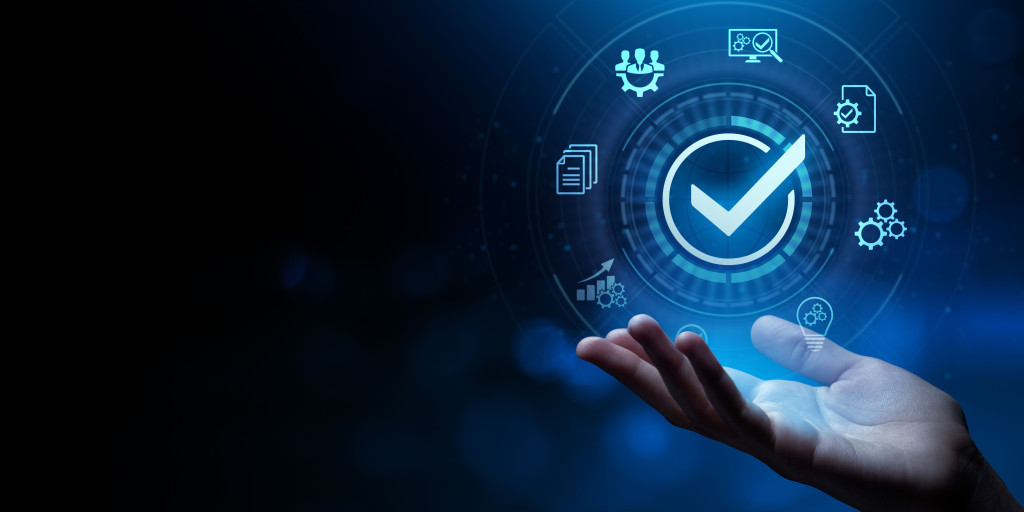