The Biggest Problems in Visual Quality Control Today
Episode 5
Author: Hanna Nennewitz
The Biggest Problems in Visual Quality Control Today
Episode 5
Author: Hanna Nennewitz
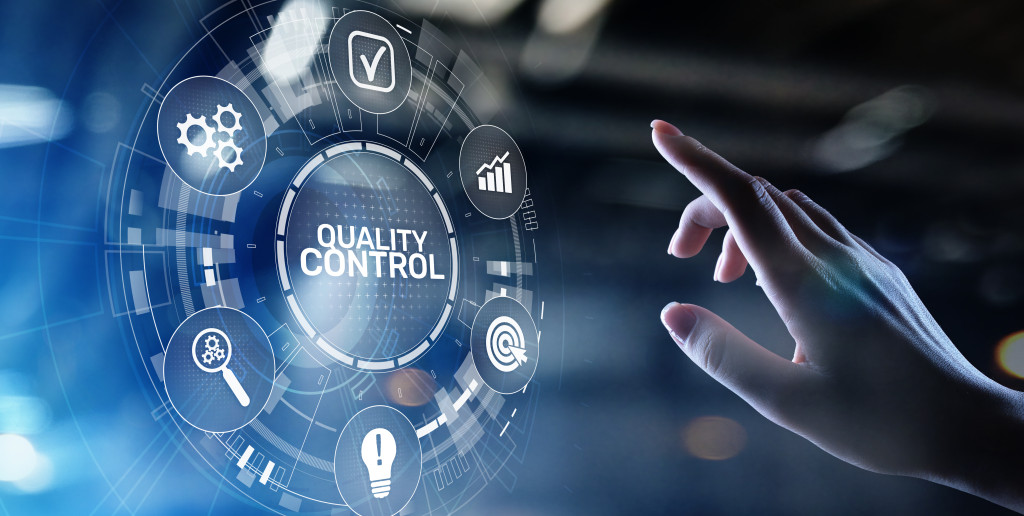
In the last few episodes, I have looked at how AI-based systems work and explained their difference from rule-based systems. Now that the workings of these systems have become clear, I turn to the question of what the biggest problems of visual quality control in industrial manufacturing are today. For that, I'm talking to Peter Droege, co-founder and CEO of Maddox AI.
In the last few episodes, I have looked at how AI-based systems work and explained their difference from rule-based systems. Now that the workings of these systems have become clear, I turn to the question of what the biggest problems of visual quality control in industrial manufacturing are today. For that, I’m talking to Peter Droege, co-founder and CEO of Maddox AI.
Hanna: Hi Peter, Dr. Wieland Brendel already explained to me in the first episode that in many companies today, visual quality control is still either rule-based or manual. The two of you founded Maddox AI together with Behar Veliqi, Prof. Dr. Matthias Bethge and Prof. Dr. Alexander Ecker because you noticed in several customer projects that this type of visual quality control causes many problems that an AI-based system can solve. What are these big problems in visual quality control today?
Peter: The problems vary depending on which quality control solution you look at. Manual control by humans has different problems than rule-based control.
Hanna: Let’s start with manual control, then. What are the biggest problems here?
Peter: The human inspector is simply not 100% attentive over a long period of time. This means that a human can easily let a mistake slip through that a machine would probably have noticed. We did tests ourselves in the team at the beginning to see how long a human can remain attentive to parts. I myself noticed a significant drop in accuracy after just 30 minutes. At some point, the work becomes very monotonous and exhausting. It can quickly happen that a faulty part slips through. In addition, we humans control very subjectively. What exactly is considered as a defect and what is not always depends on the personal opinion of the individual. For example, parts are sometimes judged differently in the morning than in the afternoon. In the same way, different examiners assess the parts differently, so that the scrap from the day depends to a large extent on the examiners used. Ultimately, one can say: If I have manual inspections today, I know by definition that I do not have particularly good sorting quality and also will send defective parts to the customer.
In addition, manual inspections are also very slow. While a machine can inspect many parts per second, humans need much longer. That makes checking with people slow and inefficient overall.
Hanna: Does Germany or Europe pose any other problems for manual inspection of products?
Peter: For all high-wage countries like Germany or even Europe as a whole, manual inspectors are always an issue. They are simply particularly expensive due to high labor costs. In addition, demographics also pose a problem for manual inspection. Due to the shortage of skilled workers, many companies can no longer find the personnel to carry out manual visual inspections. In addition, manual inspection is also a very strenuous job that a lot of people no longer want to do.
We have a total of five problems with human inspection: Checking with people is inaccurate and subjective. In addition, humans work slowly, but are associated with high labor costs in high-wage countries. Last, it is difficult to find enough people to do this work due to the shortage of skilled workers.
Hanna: Can the use of AI-based systems be seen as also helping companies in Germany to remain competitive?
Peter: You could say that. Many companies in Germany and Europe have to ask themselves how they can remain competitive in the face of rising wage costs in Germany. And there are two options here: I can either automate and thus save costs, even if I have to make an initial investment in order to continue producing in Germany. Or I can relocate production to reduce unit labor costs. In other words, automation can definitely help to secure a location.
Hanna: Earlier you made the distinction between manual control and rule-based systems. We have now talked about manual control. So, what about rule-based systems? What are the biggest issues here?
Peter: If I have very complex inspection tasks where there is a large option space of what good and bad parts look like, then rule-based systems quickly reach their limits. The production reality is often more diverse. I cannot explicitly describe this diversity with a few simple rules. You already discussed this with Dr. Wieland Brendel in episode 2. It’s easy to forget a rule or a rule doesn’t always apply and is broken. That always leads to pseudo scrap.
Hanna: Do you have a specific example where a client had this problem?
Peter: We have a customer that uses injection molding to make plastic parts. They had a huge problem with pseudo scrap, because the parts always came out of the machine slightly different, depending on the batch: The tool used to make the parts is sometimes cleaner, sometimes a little less clean, and accordingly the parts are sometimes darker, lighter, shinier, etc. They are all functional, but from a pixel perspective they all look a little different. If I then work with rigid thresholds and a rule-based system, sometimes these are broken and the parts are sorted out even though they are perfectly fine. If I want to prevent this high pseudo scrap, I have to keep recalibrating the rule-based systems on pretty much every batch. An AI-based system can learn that these differences exist. That it’s normal for parts to sometimes look a little lighter, a little darker, a little shinier, or a little less shiny. And because it has learned that these are not error characteristics, I hardly have to recalibrate the system. That means, on the one hand, I have the ability to automate very complex tasks in the first place because I know that I can model that complex task through machine learning. But I also have the advantage that I don’t have to recalibrate the system as often because I can teach the system that there are these variations in the products. That’s actually a big problem, which we hear a lot from customers who are working with rule-based systems on complex tasks. They have to recalibrate their system before almost every batch, which ends up costing a lot of effort and therefore time and money.
Hanna: Speaking of effort and time, how user-friendly are rule-based systems?
Peter: To be able to operate such a system, training is often sold along with it. These trainings are often not only two or three hours long, but sometimes extend over several days, during which you are instructed. In addition, the user interface of rule-based systems is extremely complex, especially compared to what we know from the consumer world. So, in many cases, it is also a necessity to have trained personnel just to take care of the maintenance of these systems. It’s just very complicated to operate and therefore maintain these systems.
Hanna: I found it very interesting that with the Maddox AI system, you don’t enter into a licensing agreement until we could show that the performance KPIs are being met. Did this decision also stem from a problem you guys saw in the status quo of rules-based systems?
Peter: Yes, definitely. The way visual control systems are bought today is not always in the best interest of the customer. Right now, it’s happening very much along the following logic: let’s say I buy a control system for a quarter of a million euros. Of that, I have to make a 40% down payment so that the system construction can begin. Then there might be an intermediate step in which certain functions are integrated or assembled. For this, I have to transfer another 30% of the project volume to the plant manufacturer. After that, there is an internal commissioning or an internal acceptance at the plant manufacturer’s site, where it is checked whether the plant functions properly. After that, of course, I have to make another down payment, which means that 80-90% of the project volume has already been paid. For the last 10%, the system is delivered to me as the customer for commissioning. After commissioning, I as the customer naturally check with an acceptance test whether the control system achieves the promised detection accuracy of, let’s say, 99%. However, this acceptance test reveals that the detection accuracy is only 97%. As a customer, I have then already paid 90% of the money and naturally want to use the system, even though it does not inspect as accurately as actually promised. This is not optimal from a risk logic point of view for me as a customer, since I have to invest a lot of money before I know how well the control system works. In a recent survey of industrial companies, we found that more than 40% of all companies have bought an inspection system that did not work as promised and is currently rotting in a corner of production. That is why at Maddox AI we say that the customer will not pay until we have been able to demonstrate with the system that we can deliver the promised accuracy, cycle time, etc. This means that there is no down payment with us and we bear the complete investment risk. We set up the system at the customer’s site, develop the software, fine-tune and optimize it, and the customer only pays when our control system does what was specified beforehand. If our control system doesn’t perform as promised, the customer pays nothing.
Hanna: So, the biggest problems with rule-based systems are the difficulty of automating complex inspection tasks, the low user-friendliness, and the way these systems are sold. Are there also problems that arise with both rule-based and manual inspection?
Peter: One problem that affects both types of quality control is digitalization. From our point of view, every automation project should also be a digitalization project in the end. That is, if I currently inspect manually, then I may have Excel lists or manual lists at the end of the day or week where the inspectors fill in how many defects they have found. But that is not a digital process, nor do I use that data on a large scale. At most, the data is occasionally analyzed on a project-by-project basis to determine how well or poorly production is performing at the moment at the quality level. The automatic, rule-based AOI systems that already exist have a little evaluation logic in most cases, of course, but still not much is done with the data. Yet you can gather really valuable insights with this data. For example, I can perform automatic Pareto analyses or concentration analyses. In the same way, I can be automatically notified by e-mail or SMS if I run the risk of producing too much scrap over a longer period of time. These potentials of data analysis and digitalization in the course of automation are not yet addressed to such an extent by many plant manufacturers. This is the potential we are exploiting at Maddox AI.
Hanna: You’ve already suggested that AI-based systems are an important way to solve the biggest problems in visual quality control. But why is it taking so long for AI-based systems to become mainstream in the industry? After all, as consumers we’ve been using AI-based software on our cell phones, for example, for quite a while.
Peter: I think AI-based systems have been around for quite a while in the industry, and I think the marketing for AI systems in particular is relatively big. Nevertheless, these systems don’t take off as quickly in the industry as AI systems do in the consumer world. I think that can be explained mainly by data accessibility. In the B2C space, I just have millions, billions of data points of images, voice, etc. freely available over the Internet. You can do a lot with that data, of course. Data in an industry context is harder to get because most companies are afraid to share data. In other words, the data that you need to develop AI systems for industrial use was not freely available and, accordingly, it took much longer to develop AI-based systems for industry. So, the B2C world had a natural head start, so to speak, because the data was much more available.
A second issue, I think, is the attractiveness of applications. This is simply higher for some applications than for others. Let’s take a look at advertising, for example. That’s where Facebook uses AI algorithms to play out ads. And the “vertical” advertising is, of course, a big topic with a lot of value in it. At the same time, all I need for that is one AI model or one AI model family to play out those ads. So, I have a huge scope, but have relatively little effort to get a lot of value. Because the opportunity/cost ratio is so attractive, larger amounts of money have been quickly invested in this area, which has rapidly driven the development and proliferation of AI systems. The same is true for Google’s search engine, for example. Again, I can create quite a bit of value with one model, one family of models.
Hanna: And what does that look like in terms of visual quality control?
Peter: Here we have a much bigger long tail. There are many smaller use cases. In these use cases, I can see that, for example, visual inspection of wire, seals or even vehicles are a relevant topic, but only for a smaller customer base in each case. This means that I have to solve many individual modeling tasks, all of which are worthwhile and meaningful. The market is therefore much smaller than the advertising or search market. Across all use cases, however, it is still huge, of course.
So, in summary, on the one hand, I don’t have the data at all, or I have very little data, to develop AI-based control systems. On the other hand, the added value I can achieve with a single model is significantly less than with a super good search algorithm. In the visual quality control space, it was therefore necessary to build a platform that allows companies with their individual use cases to develop a good AI model even for their more specific use cases. This is exactly the platform we built with Maddox AI.
Hanna: Thank you Peter, that was very insightful.
If you found yourself in these problems, it makes sense to think about an AI-based control system. Feel free to contact us, we are happy to check if the Maddox AI system can reliably automate quality control for you as well.
In the next episodes, I will take a closer look at the benefits of AI-based systems in industrial applications and again talk to Peter Droege to find out whether AI-based control systems are also financially worthwhile for companies.
Why invest in AI for quality control? - A sample calculation.
Episode 6
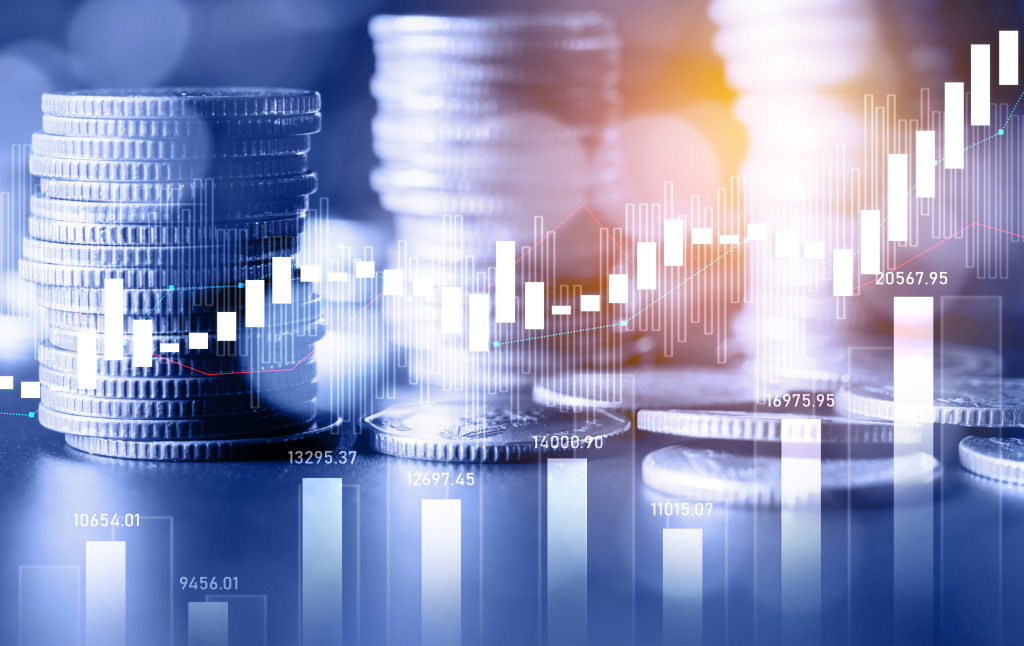
By investing in an AI-based inspection system, five financial levers can be turned. In this blog post, you will learn why and how exactly an AI-based inspection system has an influence on these levers.
Read the article